Artificial intelligence is here and software development will never be the same… or will it? The advancements made in training AI models to accomplish various tasks are nothing short of astounding, but the appeal of these shiny new tools is leading companies of all sizes to imagine what their own bespoke AI could look like.
With pleasant dreams of seamlessly integrating a do-it-all machine learning model — built in-house from scratch — the C-Suite decides that breakthroughs have to happen within their own walls, and lofty budgets are allocated as a result.
The truth is this doesn’t have to happen, and for the vast majority of companies, it probably shouldn’t. With widespread availability of suitable AI frameworks available, there’s virtually no reason why most companies should consider building their own artificial intelligence.
Reality Check
There are endless ways for a company to harness the power of artificial intelligence. From monitoring the supply chain to industry analysis and even interacting with customers, choosing how and when to add AI to your company’s overall strategy is part of what Vincit does every day.
But there is a growing desire among mid-sized companies to build their own AI models from the ground-up. It’s a trend born out of an overzealous approach to technological breakthroughs and at its core is a belief that for a technology to work well, it has to be built for a singular purpose. For some technologies, this is actually true. If you’re in shipping, you wouldn’t want a vehicle meant to haul cargo to be built on the same frame as a Volkswagen Beetle and then customized afterwards, right?
Thankfully, artificial intelligence is much different, and a healthy and responsible integration strategy will allow a company to leverage existing AI models in powerful ways, and with far fewer headaches than would come with building a model from scratch.
Two of the biggest misunderstandings about AI within the business world are actually on opposite ends of the spectrum. Some believe that AI foundations are built for one use case and one use case only, while others have been misled to believe that any AI model can do just about anything, as long as it’s had enough modification.
The truth is somewhere in the middle. Yes, many AI frameworks are powerful and flexible, but none are great at everything. This is where some companies run into problems when making the decision about how to integrate the technology.
Depending on the use case, there are a number of approaches that can be taken.
For example, an email marketing company might use a completely off-the-shelf or SaaS option, like ChatGPT, to give their employees a starting point for outreach campaigns, requiring no in-house development or tweaking whatsoever. In this case, there’s no need to develop or even tweak anything, as long as employees know how to use the tool the right way.
Meanwhile, a company that wishes to integrate AI to take some of the weight off of its customer service representatives’ shoulders could easily use an open source conversational model and adjust it as necessary to respond to customers’ needs. This would require more work than an SaaS option, but significantly less work than building an in-house AI from scratch.
However, a company that needs an AI model to perform a very specific task — like monitoring the efficiency and predictive maintenance routines of a machine or process built by that same company — may opt to build an AI foundation from scratch to ensure ultimate accuracy. This would require in-depth knowledge and development of the AI within the organization or by a trusted software partner.
Wrong Direction
It’s easy to see the appeal of building a custom AI. It’s an exciting new technology, and everyone wants to feel like they’re on the absolute cutting edge. Realistically, building an AI is more likely to put you behind your competitors than ahead of them.
Imagine two companies, which we’ll call Company A and Company B. Company A decides to build its own AI in-house and Company B decides to adopt an existing model that has been customized to suit its needs using an existing data set that provides the artificial intelligence with enough information to pull from for reliably accurate results.
It might take six months (a conservative estimate here) for Company A to choose an AI framework, collect and clean a data set, train the AI to create a model, test it for errors, and eventually implement it. In that time, Company B not only integrated its customized AI, but they’ve trained their staff to use it effectively and are better prepared for whatever comes next.
Even more worrisome, the odds of an unexpected issue popping up for the first time in a new AI model are an order of magnitude greater than the same happening with a tried and true model. Simply put, however much testing an in-house AI gets before going live, it’s likely not enough.
The Responsible and Cost Effective Approach
By now, you no doubt understand the drawbacks of building an in-house AI, not to mention the associated costs of such an approach, both in terms of time and money. So, why would anyone decide that they needed their very own AI?
There are a few reasons: A company’s leadership team might have a fundamental misunderstanding of how an AI model works, or are unwilling to learn. Technology teams within mid-sized companies might have their own motives for insisting on building an AI from scratch, too. There’s also the undeniable fact that having your own AI — rather than using an existing model — may be seen as a sort of industry status symbol.
But at the end of the day, none of this matters to your employees or customers who will be interacting with the AI on a day-to-day basis. They just want something that works, and works well, and mid-sized companies would do well to strongly consider integrating an existing AI model rather than building their own.
Contact us to discuss more!
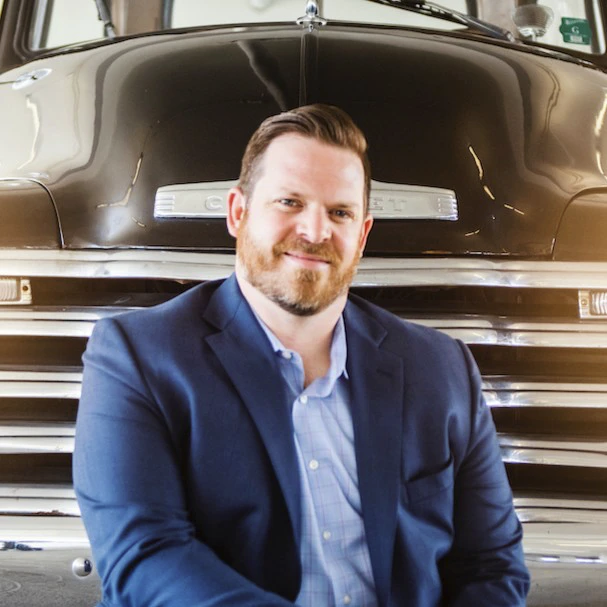
Sean Richards,
Vincit Alumn